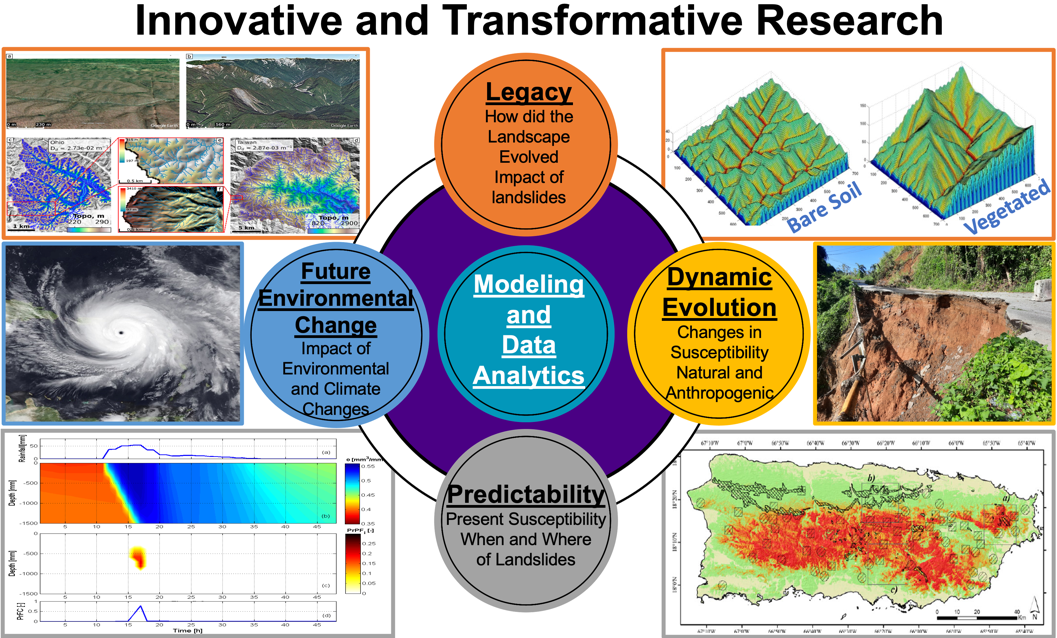
Introduction
Innovative and Transformative Research is the science core of the CCLG. The research efforts consist of the five thrusts introduced above and shown in the Figure: (1) Legacy, (2) Dynamic Evolution, (3) Predictability, (4) Future Environmental Change, and (5) Modeling and Data Analytics.
The CCLG will contribute to landslide hazard science research in two ways.
1) Center participants will address a specific set of focused questions of importance to each region. Although these questions may address specific sites or events, we approach the science with the goal of formulating a general theoretical understanding that transcends any single region and can, for example, explain why, in one setting, debris flows are common while another is prone to deep-seated failures.
2) More broadly, the CCLG will contribute to supporting research on the above questions across the wider landslide science community. Our approach builds on the premise articulated above (Premise 2) that by coordinating the national landslide hazard science community, the Center will catalyze and accelerate progress in landslide research.
CCLG investigators will be guided by the questions posed but can be only expected to be an important part of a much larger effort required to address these research challenges. CCLG is not just about its existing scientists but about bringing together a community. To help catalyze broad research endeavors, the CCLG will invite proposals from the community that address the science questions of interest to the Center. Seed funding of $100,000 a year has been budgeted for this purpose. The intention is to fund pilot efforts with the potential for large returns and leveraging of additional resources. This opportunity will be open to members of the landslide hazard science community (excluding CCLG Institutions) to explore landslide hazard science topics, including the Education and Workforce Development and Community Engagement and Broadening Participation MAs described below.
The overarching goal of the Innovative and Transformative Research Major Activity is to: deepen the fundamental understanding of landsliding by integrating the rapidly growing volume and quality of relevant data with a community-based landslide modeling framework that enables rapid hypothesis testing and refinement.
Background
There is an ever-increasing volume and quality of environmental data for topography, soils, lithology, ecosystems, land cover and land use change, hydrology, climate, and seismicity developed from long-term local observations, remote sensing platforms, and hybrid products that combine observational and modeled data. Yet the development of new theories and physical models of the Earth’s surface has been limited.
We rely on a handful of analytical theories for landslide initiation that are based on force balance on a failure plane and different geometries and scales. Although these approaches may be applicable under idealized and uniform soil conditions, they are less adequate within a complex matrix of soil texture, plant root systems, hydrologic pathways, and lithologic controls, and there exists a scaling mismatch between these approaches and regional-scale risk assessment.
More importantly, there is no place in these traditional formulations to parameterize and use the wealth of information available to us. Recently, a growing number of studies have used data-driven statistical and machine learning (ML) methods to investigate the relative contributions of environmental conditions, along with climatic forcing, on landslide patterns. Nevertheless, ML models remain challenging to interpret, may not capture causal relationships or sensitivities, and may not extrapolate outside of the range of training data. The joint use of data-driven tools and mechanistic models in ways that leverage the fundamental strengths of ML is an area ripe for development .
Disturbances such as timber harvesting, land conversion, wildfires, and tree die-offs can directly or indirectly increase landslides and runoff-driven debris flows for a period, but mechanistic theory or empirical models are not well suited to incorporate the dynamic effects of such surface disturbances. Infrastructure such as roads, and urbanization have effects on the environment, influencing landslide risk, but most existing models often oversimplify how the built environment interacts with hydrology and soil states.
Research Plan
As we emphasized in the Figure, a landslide we observe today (or risks we predict into the future) is the compound outcome of a particular triggering event with pre-existing landscape conditions and/or anthropogenic modifications. Similarly, the occurrence of future landslides will depend on geologic and human legacies in combination with current and future trends in climate and land use. To advance landslide hazard science and improve models for landslide initiation, we should move away from disciplinary silos to truly collaborative, inclusive, adaptive, and interactive methods, where we can ask “out of the box” questions as a community, and systematically pose new hypotheses, and develop new theories and models. To assist in this collaborative science, there is an urgent need to move landslide research to cyber platforms where environmental data are curated and seamlessly integrated with physically based, data-driven, and hybrid modeling approaches that meet user community needs.
To meet this challenge, the CCLG will build and implement a Landslide Modeling Portal for connecting to the curated site-specific datasets that comprise Community Landslide Virtual Observatories (CLaVOs). The Landslide Modeling Portal will provide a library of mechanistic and data-driven models and model coupling tools, with capabilities for transferring geospatial and time series data from existing platforms like Hydroshare, OpenTopography, and Earth observation satellites. CLaVOs will be designed for use with the Landslide Modeling Portal to make community landslide research easier, more equitable, and inclusive. Data science tools for creating CLaVOs will be developed for the community to start their own virtual observatories. The proposed cyberinfrastructure will create an opportunity to activate a “learning loop”: an iterative comparison of data and theory (models) that leads to a progressively deeper understanding of landslides within the complex earth surface system.